Integration use case: Performing analysis on immuneSIM-generated repertoires
This use case will show you how to use immuneML in conjunction with immuneSIM (Weber et al. 2020), tunable multi-feature simulation tool of B- and T-cell receptor repertoires for immunoinformatics benchmarking.
The combined use of these tools enables the user to generate datasets with know signals as a baseline for ML classification. The user can either input already existing immuneSIM repertoires into the workflow or use a cross-platform metadata file to both simulate datasets and provide the label information for immuneML input.
For reference, a detailed documentation of immuneSIM including an installation guide can be found here. Additionally, example files for this particular workflow are available on the immuneSIM GitHub. Note: This use case is based on immuneSIM v0.9.0.
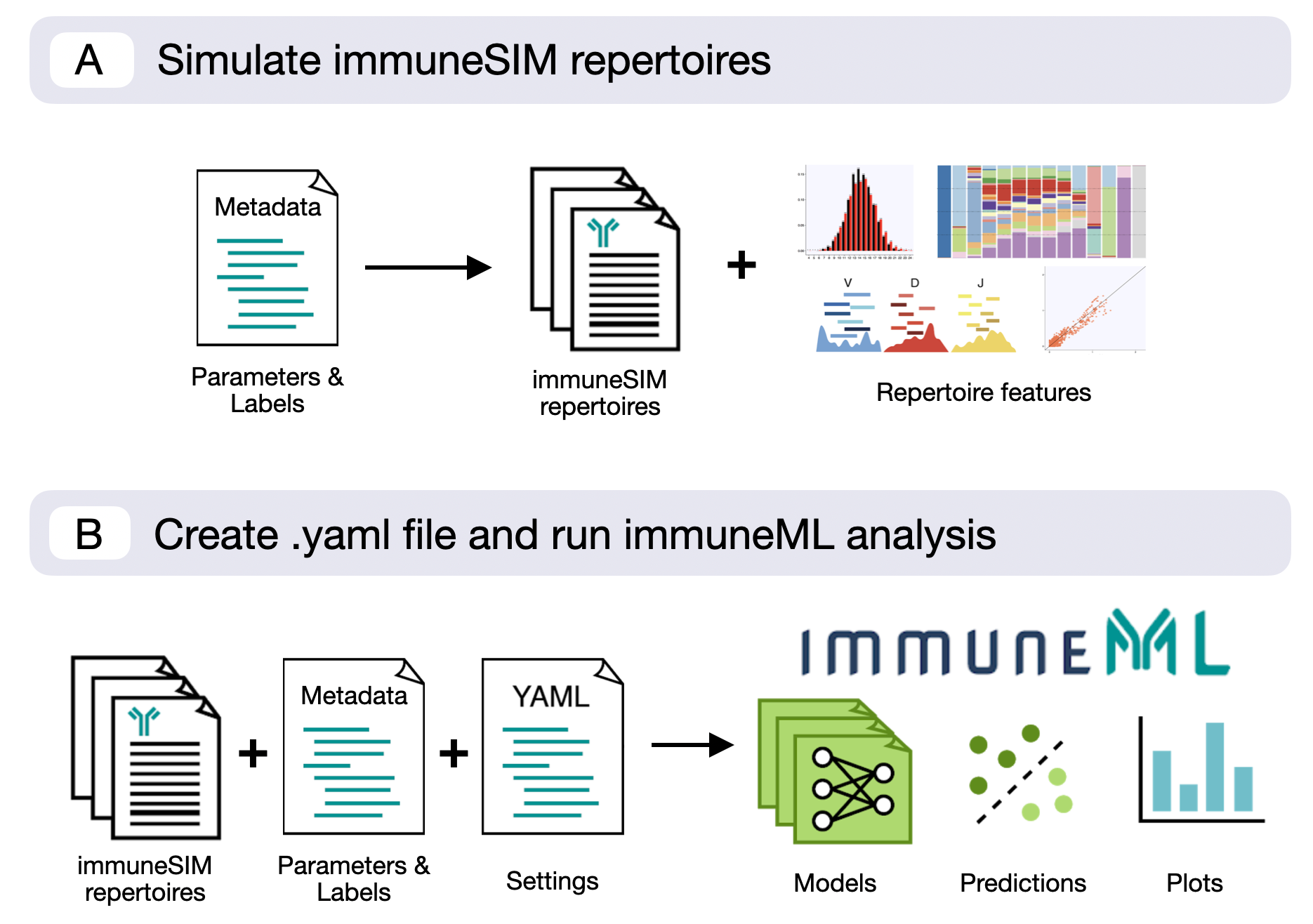
Combined workflow with an immuneSIM and immuneML compatible metadata file
The most efficient way to combine immuneSIM and immuneML is by using a single metadata file for both the simulation in immuneSIM and training the model in immmuneML. Below an example of a metadata table, containing parameters for the simulation of immuneSIM repertoires, which can also be used as metadata in an immuneML workflow.
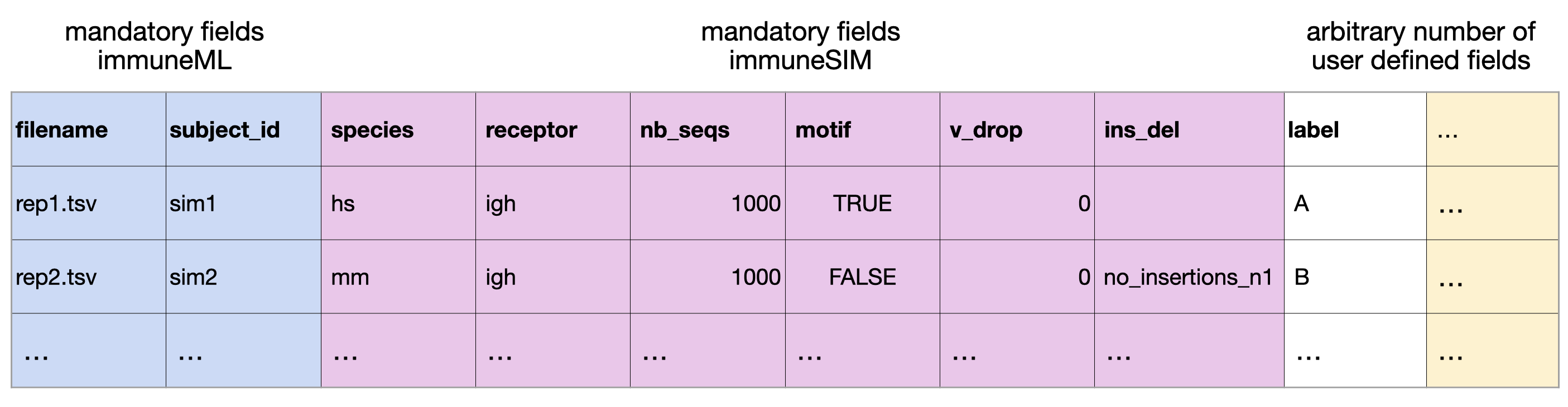
This metadata file (metadata_full_sim.csv
) is used by the following immuneSIM script to generate a set of repertoires.
The metadata file can then be fed into immuneML together with the resulting simulated repertoires
as described in the How to import data into immuneML section.
The following R script generates the simulated repertoires using immuneSIM. The script can also be downloaded here: immuneSIM_for_ML.R
.
## ImmuneML use case (https://immuneml.uio.no/)
# This script simulates immuneSIM repertoires based on an immuneML compatible metadata file.
# requires immuneSIM 0.9.0 (github: https://github.com/GreiffLab/immuneSIM)
library(immuneSIM)
PATH <- "./immuneML_Sim"
#load metadata file
metadata <- read.delim(file.path(PATH,"metadata_full_sim.csv"),sep=",")
#Define motif for cases where motif==TRUE. Here two motifs are inserted with a probability of 0.5 at a fixed position.
motif <- data.frame(aa=c("AA","FF"),nt=c("gccgcc","tttttt"),freq=c(0.5,0.5))
fixed_pos <- 4
#for each line in metadata simulate a repertoire and write out.
for(i in 1:nrow(metadata)){
#simulate repertoire
curr_df <- immuneSIM(number_of_seqs = metadata$nb_seqs[i],
vdj_list = list_germline_genes_allele_01,
species = metadata$species[i],
receptor = substr(metadata$receptor[i],1,2),
chain = substr(metadata$receptor[i],3,3),
insertions_and_deletion_lengths = insertions_and_deletion_lengths_df,
user_defined_alpha = 2,
name_repertoire = metadata$filename[i],
length_distribution_rand = length_dist_simulation,
random = FALSE,
shm.mode = 'none',
shm.prob = 15/350,
vdj_noise = 0,
vdj_dropout = c(V=metadata$v_drop[i],D=0,J=0),
ins_del_dropout = metadata$ins_del[i],
equal_cc = FALSE,
freq_update_time = round(0.5*metadata$nb_seqs[i]),
max_cdr3_length = 100,
min_cdr3_length = 6,
verbose = TRUE,
airr_compliant = TRUE)
#after simulation implant motifs
if(metadata$motif[i]==TRUE){
curr_df <- motif_implantation(curr_df, motif,fixed_pos)
}
#write repertoire to file
write.table(curr_df,file=file.path(PATH, "data", metadata$filename[i]),sep="\t",quote=FALSE,row.names=FALSE)
}
Using existing immuneSIM repertoires with immuneML
As immuneSIM repertoires use AIRR-compliant column naming, they can be directly fed into any immuneML workflow using AIRR importer. For this, a metadata file indexing the existing immuneSIM repertoires and indicating classification relevant labels has to be created.
If the user chooses to write their own using .yaml file, the declaration of format: AIRR
in the definition section is sufficient to ensure compatibility with immuneSIM datasets.
Here we show an example of the analysis using immuneSIM-generated repertoires to train a logistic regression and examine the coefficients of the model.
definitions:
datasets:
immuneSIM_dataset: # user-defined dataset name: here described the immuneSIM dataset
format: AIRR
params:
path: ./immuneML_Sim/data/ # path to the folder containing the repertoire files generated by immuneSIM
metadata_file: ./immuneML_Sim/metadata_full_sim.csv
encodings:
my_kmer_frequency: # user-defined encoding name
KmerFrequency: # encoding type
k: 3 # encoding parameters
ml_methods:
my_logistic_regression: LogisticRegression # user-defined ML model name: ML model type (no user-specified parameters)
reports:
my_coefficients: Coefficients # user-defined report name: report type (no user-specified parameters)
instructions:
my_training_instruction: # user-defined instruction name
type: TrainMLModel
dataset: immuneSIM_dataset # use the same dataset name as in definitions
labels:
- label # use a label available in the metadata file
settings: # which combinations of ML settings to run
- encoding: my_kmer_frequency
ml_method: my_logistic_regression
assessment: # parameters in the assessment (outer) cross-validation loop
reports: # plot the coefficients for the trained model
models:
- my_coefficients
split_strategy: random # how to split the data - here: split randomly
split_count: 1 # how many times (here once - just to train and test)
training_percentage: 0.7 # use 70% of the data for training
selection: # parameters in the selection (inner) cross-validation loop
split_strategy: random
split_count: 1
training_percentage: 1 # use all data for training
optimization_metric: balanced_accuracy # the metric to optimize during nested cross-validation when comparing multiple models
metrics: # other metrics to compute for reference
- auc # area under the ROC curve
- precision
- recall